What is data enrichment ? Data enrichment is the process of enhancing existing information by merging it with additional external data, improving data quality and value.
Data enrichment is a process of enhancing and refining raw data to make it more useful and valuable for businesses. It involves adding new data elements, correcting inaccuracies, and removing duplicates to create a more complete and accurate database. Data enrichment is becoming increasingly important in today’s data-driven world, where businesses rely on data to make informed decisions.
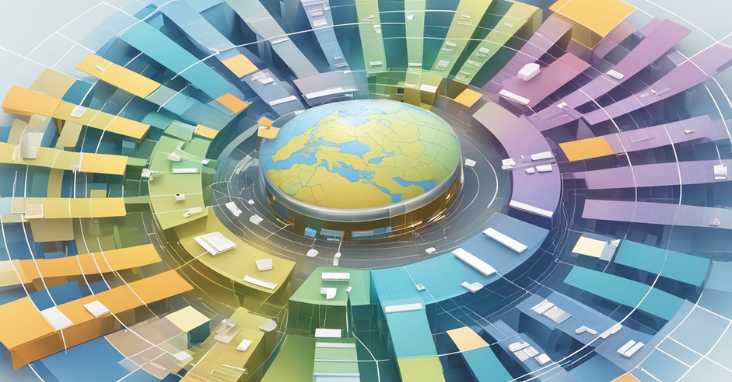
Understanding data enrichment is crucial for businesses that want to maximize the value of their data. It involves identifying the sources of data, collecting and consolidating it, and then applying various techniques to enrich it. Data enrichment can help businesses gain insights into customer behavior, market trends, and business performance, which can be used to drive growth and profitability. By enriching their data, businesses can gain a competitive edge and make better decisions based on accurate and relevant information.
Key Takeaways
- Data enrichment is the process of enhancing and refining raw data to make it more useful and valuable for businesses.
- Understanding data sources and collection, data quality and cleansing, and data enrichment techniques is crucial for successful data enrichment.
- Data enrichment can help businesses gain insights into customer behavior, market trends, and business performance, which can be used to drive growth and profitability.
Understanding Data Enrichment
Definition and Importance
Data enrichment is the process of enhancing, refining, or improving raw data by adding additional information to it. This additional information can include demographic data, geographic data, financial data, and more. The importance of data enrichment lies in the fact that it can greatly enhance the value of data by making it more accurate, complete, consistent, and relevant.
Data enrichment is often used in marketing, sales, and customer service to gain a better understanding of customers and prospects. By enriching customer data with additional information such as their demographic data, interests, and purchasing behavior, businesses can better target their marketing efforts and provide more personalized customer experiences.
Key Components of Data Enrichment
The key components of data enrichment include accuracy, value, quality, completeness, consistency, and relevance. Accuracy refers to the correctness of the data, while value refers to the usefulness of the data. Quality refers to the reliability and validity of the data, while completeness refers to the extent to which all relevant data is included. Consistency refers to the uniformity of the data, while relevance refers to the importance of the data to the intended purpose.
To ensure the success of data enrichment, it is important to have a clear understanding of the key components and to use reliable data sources. One such reliable data source is the US Census Bureau, which provides a wealth of demographic data that can be used for data enrichment purposes.
For more information on data enrichment, check out this resource.
Data Sources and Collection
Primary Data Sources
Primary data sources refer to the data that a company collects directly from its customers or users. This type of data is considered first-party data, and it can include information such as customer demographics, purchase history, and website usage patterns. Companies can collect primary data through various methods such as online surveys, feedback forms, and user registration forms.
Using Third-Party Data
In addition to primary data sources, companies can also use third-party data to enrich their existing data sets. Third-party data refers to data that is collected by external data sources such as data brokers, social media platforms, and public records. This data can provide valuable insights into consumer behavior, interests, and preferences. Companies can use third-party data to enhance their customer profiles and create more targeted marketing campaigns.
One popular source of third-party data is social media platforms. Companies can use social media data to gain insights into their customers’ interests, preferences, and behaviors. For example, a company that sells outdoor gear may use social media data to identify customers who are interested in hiking, camping, and other outdoor activities.
Data Collection Methods
Data collection methods can vary depending on the type of data being collected. For example, companies may use online surveys to collect customer feedback or use website tracking tools to monitor user behavior. Other data collection methods include focus groups, in-person interviews, and customer loyalty programs.
It is important for companies to ensure that they are collecting data in a responsible and ethical manner. This includes obtaining proper consent from customers and ensuring that the data is secure and protected. Companies should also be transparent about their data collection practices and provide customers with clear information about how their data is being used.
To learn more about data enrichment and best practices for data collection, check out this resource on Data Enrichment from HubSpot.
Data Quality and Cleansing
Data quality and cleansing are crucial components of data enrichment. Inaccurate data can lead to incorrect analysis, ineffective decision-making, and lost opportunities. Data quality issues can arise from various sources, including human error, system glitches, and outdated information. Therefore, ensuring data accuracy and maintaining data hygiene are essential for effective data enrichment.
Ensuring Data Accuracy
To ensure data accuracy, it is essential to establish data quality standards and guidelines. These standards should include data validation rules, data profiling, and data auditing. Data validation rules ensure that data is entered correctly and consistently, while data profiling identifies data quality issues and inconsistencies. Data auditing involves regular checks to ensure that data quality standards are being met and maintained.
Data Cleansing Techniques
Data cleansing involves identifying and correcting errors and inconsistencies in data. There are various data cleansing techniques, including data parsing, data standardization, and data enrichment. Data parsing involves breaking down data into smaller components, while data standardization involves converting data into a consistent format. Data enrichment involves adding missing data to existing data sets to improve their value and accuracy.
Maintaining Data Hygiene
Maintaining data hygiene involves ensuring that data is up-to-date, consistent, and free from errors. Data hygiene practices include regular data cleansing, data profiling, and data validation. Data cleansing involves identifying and correcting errors and inconsistencies in data, while data profiling identifies data quality issues and inconsistencies. Data validation ensures that data is entered correctly and consistently.
To maintain data hygiene, it is crucial to establish data quality standards and guidelines, conduct regular data audits, and invest in data cleansing tools and technologies. By ensuring data accuracy and maintaining data hygiene, organizations can enhance their data enrichment efforts and derive meaningful insights from their data.
For more information on data quality and cleansing, check out this resource.
Data Enrichment Techniques
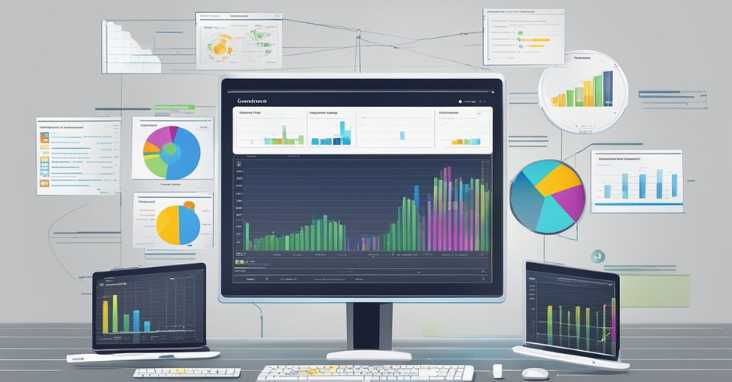
Data enrichment techniques are used to enhance the quality and completeness of existing data. These techniques involve the use of various tools and methods to append, transform, integrate, and impute data. In this section, we will discuss some of the commonly used data enrichment techniques.
Enrichment Tools and Automation
Enrichment tools and automation refer to the use of software tools and automated processes to enrich data. These tools can help to streamline the process of data enrichment and reduce the risk of errors. Some of the popular enrichment tools include:
- Google Refine: This tool can be used to clean and transform data, and to reconcile data from different sources.
- OpenRefine: This tool can be used to clean and transform data, and to reconcile data from different sources.
- Talend: This tool can be used for data integration, data quality, and data management.
Data Appending and Transformation
Data appending and transformation involve adding new data to existing data and transforming data into a standardized format. These techniques can help to improve the accuracy and completeness of the data. Some of the popular data appending and transformation methods include:
- Address standardization: This involves standardizing addresses to a common format, which can help to eliminate duplicates and improve accuracy.
- Name parsing: This involves breaking down names into their component parts, which can help to improve accuracy when matching records.
- Email validation: This involves verifying the validity of email addresses, which can help to eliminate invalid records.
Data Integration and Imputation
Data integration and imputation involve combining data from multiple sources and filling in missing values. These techniques can help to improve the completeness and accuracy of the data. Some of the popular data integration and imputation methods include:
- Record linkage: This involves linking records from different sources based on common attributes, such as name and address.
- Data imputation: This involves filling in missing values using statistical methods, such as regression analysis.
- Data fusion: This involves combining data from multiple sources to create a single, comprehensive dataset.
Overall, data enrichment techniques can help to improve the quality and completeness of data, which can lead to better decision-making and improved business outcomes. To learn more about data enrichment techniques, check out this resource.
Data Enrichment Applications
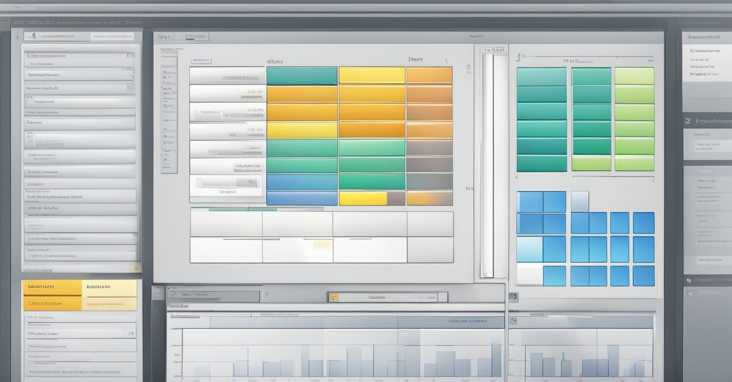
Data enrichment is a powerful tool that can help businesses gain valuable insights into their customers and improve their overall customer experience. By enhancing customer profiles, targeting and personalization, and improving customer engagement, businesses can create more personalized and effective marketing campaigns.
Enhancing Customer Profiles
One of the key benefits of data enrichment is the ability to enhance customer profiles. By collecting and analyzing data from various sources, businesses can gain a more complete picture of their customers, including their demographics, interests, and behaviors. This information can be used to create more targeted and personalized marketing campaigns that resonate with customers on a deeper level.
Targeting and Personalization
Data enrichment can also help businesses improve their targeting and personalization efforts. By analyzing customer data, businesses can identify patterns and trends that can be used to create more effective marketing campaigns. For example, businesses can use data to identify customers who are most likely to make a purchase and target them with personalized offers and promotions.
Improving Customer Engagement
Finally, data enrichment can help businesses improve their overall customer engagement. By understanding customer behavior and preferences, businesses can create more personalized and engaging experiences that keep customers coming back. For example, businesses can use data to personalize customer interactions, such as recommending products or services based on past purchases.
Overall, data enrichment is a powerful tool that can help businesses improve their customer relations and create more personalized customer experiences. By leveraging customer data, businesses can gain valuable insights into customer behavior and preferences, and use this information to create more effective marketing campaigns. For more information on data enrichment and its applications, check out this external resource.
Data Enrichment in CRM
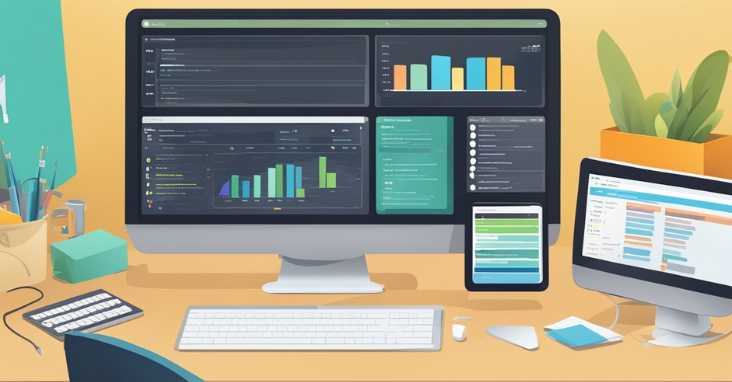
Data enrichment is a process that involves enhancing existing data with additional information to improve its accuracy and value. In CRM, data enrichment can help businesses gain a deeper understanding of their customers and prospects, which in turn can lead to more effective marketing and sales efforts.
Integrating Enriched Data into CRM
Integrating enriched data into CRM can help businesses get a more complete picture of their customers. By combining data from different sources, businesses can gain insights into customer behavior, preferences, and needs. This can help businesses tailor their marketing and sales efforts to better meet the needs of their customers.
One of the most popular CRM platforms is HubSpot CRM. HubSpot CRM offers a range of features that can help businesses integrate enriched data into their CRM. For example, HubSpot CRM allows businesses to import data from different sources, such as spreadsheets or other CRM systems. This data can then be enriched using HubSpot’s data enrichment tools, which can help businesses fill in missing information about their customers.
CRM Data Enrichment Best Practices
To get the most out of data enrichment in CRM, businesses should follow some best practices. Here are a few tips:
- Start with valid data: Before enriching your data, make sure it is accurate and up-to-date. Invalid data can lead to inaccurate enrichments, which can be detrimental to your marketing and sales efforts.
- Use multiple data sources: To get the most complete picture of your customers, use data from multiple sources. This can include social media, web analytics, and other CRM systems.
- Prioritize data: Not all data is created equal. Prioritize the data that is most important to your business goals, and focus your enrichment efforts on that data.
- Keep data protection in mind: When enriching your data, make sure you are following data protection regulations. This can include obtaining customer consent and storing data securely.
To learn more about data enrichment in CRM, check out this article by Salesforce, a leader in CRM software.
Analyzing Enriched Data
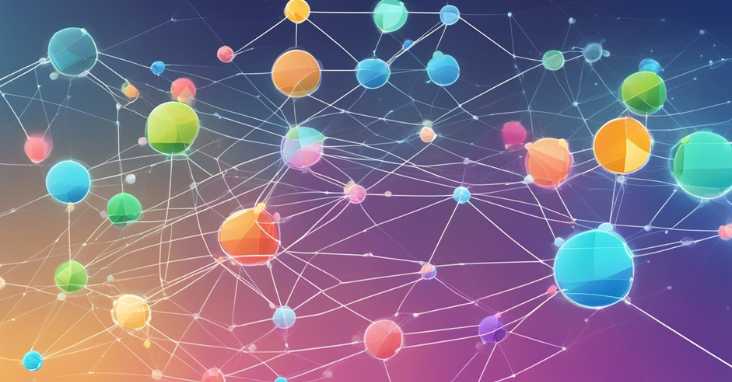
After the data has been enriched, the next step is to analyze it. This section will cover two important aspects of analyzing enriched data: Business Intelligence Insights and Data-Driven Decision Making.
Business Intelligence Insights
Business Intelligence (BI) is the process of analyzing data to provide insights that can help businesses make better decisions. Enriched data can be used to generate BI insights that were not possible before. For example, a dataset enriched with demographic data can be used to identify patterns and trends in customer behavior based on age, gender, and income.
To generate BI insights, data analysts use various techniques such as data visualization, data mining, and statistical analysis. These techniques help to identify patterns and trends in the data that can be used to make informed decisions. For instance, data visualization can be used to create interactive dashboards that provide a real-time view of key performance indicators.
Data-Driven Decision Making
Data-Driven Decision Making (DDDM) is the process of making decisions based on data rather than intuition or personal experience. Enriched data can be used to make data-driven decisions that were not possible before. For example, a dataset enriched with social media data can be used to identify customer sentiment and preferences.
To make data-driven decisions, businesses need to have a clear understanding of the data they have and how it can be used to solve business problems. Machine learning algorithms can be used to analyze the data and identify patterns and trends that can be used to make informed decisions. These decisions can then be translated into actionable insights that can be used to improve business processes and outcomes.
In conclusion, analyzing enriched data is an important step in the data enrichment process. Business Intelligence insights and Data-Driven Decision Making are two important aspects of analyzing enriched data that can help businesses make better decisions. By using various techniques such as data visualization, data mining, and statistical analysis, businesses can identify patterns and trends in the data that can be used to generate actionable insights. For more information on data enrichment, check out this link.
Compliance and Data Governance
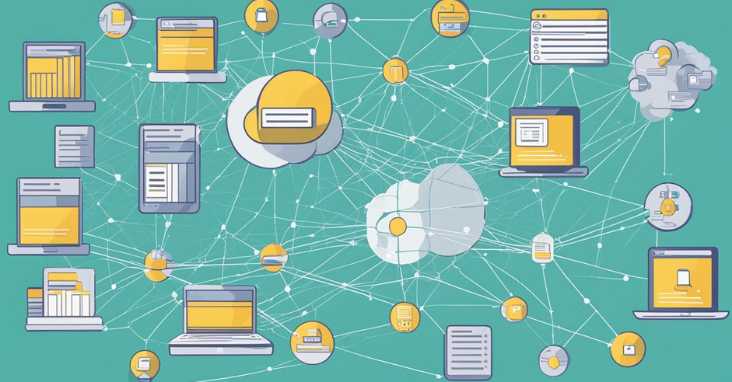
Data enrichment is an ongoing process that requires compliance with various regulatory frameworks and data governance practices. Compliance and data governance are critical aspects of data enrichment, as they ensure that the data being enriched is accurate, reliable, and secure.
Regulatory Compliance
Regulatory compliance is a critical aspect of data enrichment. Compliance ensures that the data being enriched meets the standards set by various regulatory frameworks, such as GDPR, CCPA, and HIPAA. These frameworks have set strict guidelines for data privacy and security, and failure to comply with these regulations can result in severe penalties and legal action.
To ensure regulatory compliance, organizations must implement robust data governance practices that cover data collection, storage, processing, and sharing. They must also have a clear understanding of the data they are enriching, including its source, quality, and accuracy.
Risk Management and Data Integrity
Risk management and data integrity are also crucial aspects of data enrichment. Risk management involves identifying and mitigating potential risks associated with data enrichment, such as data breaches, cyber attacks, and data loss. Data integrity, on the other hand, ensures that the enriched data is accurate, complete, and consistent.
To ensure risk management and data integrity, organizations must implement robust data governance practices that cover data security, access control, and data quality. They must also have a clear understanding of the risks associated with data enrichment and have measures in place to mitigate these risks.
Overall, compliance and data governance are critical aspects of data enrichment that ensure the accuracy, reliability, and security of enriched data. Organizations must implement robust data governance practices and comply with various regulatory frameworks to ensure the integrity of the enriched data.
Here is a link to an external resource that provides more information on data governance and compliance.
Advancing with Data Enrichment
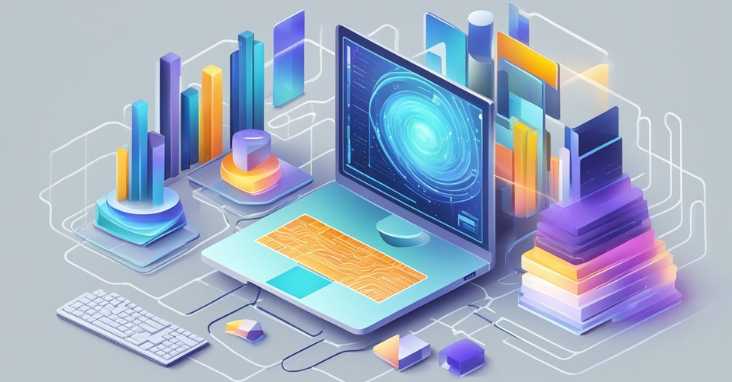
Data enrichment is a powerful tool that enables businesses to gain insights into their customers and make informed decisions. By adding relevant data to existing customer information, businesses can better understand their customer base and tailor their marketing efforts accordingly. Here are some of the ways businesses can advance with data enrichment.
Future Trends in Data Enrichment
As technology continues to evolve, data enrichment is becoming more sophisticated and powerful. In the future, businesses can expect to see more advanced algorithms and machine learning techniques that will enable them to gain even deeper insights into their customers. This will allow businesses to make more informed decisions about their marketing efforts, product development, and business strategy.
Continuous Improvement and Adaptation
To stay competitive in today’s fast-paced business environment, businesses must be able to adapt quickly to changing market conditions. Data enrichment can help businesses stay ahead of the curve by providing them with real-time insights into customer behavior and market trends. By continuously improving and adapting their data enrichment strategies, businesses can stay ahead of their competitors and make more informed decisions.
One example of how data enrichment can be used is to segment customers based on demographic and psychographic information. By understanding their customers’ needs and preferences, businesses can tailor their messaging and marketing efforts to better resonate with their target audience. This can lead to increased revenue and customer loyalty.
Another example is the use of weather data to inform business decisions. By analyzing weather patterns and their impact on customer behavior, businesses can adjust their marketing efforts and product offerings accordingly. For example, a clothing retailer could use weather data to promote raincoats and umbrellas during a rainy season, or sunscreen and beachwear during the summer.
In conclusion, data enrichment is a powerful tool that can help businesses make informed decisions and stay ahead of their competitors. By continuously improving and adapting their data enrichment strategies, businesses can gain deeper insights into their customers and make more informed decisions about their marketing efforts, product development, and business strategy. To learn more about data enrichment, check out this [link to an external resource with high authority on the topic].
Frequently Asked Questions
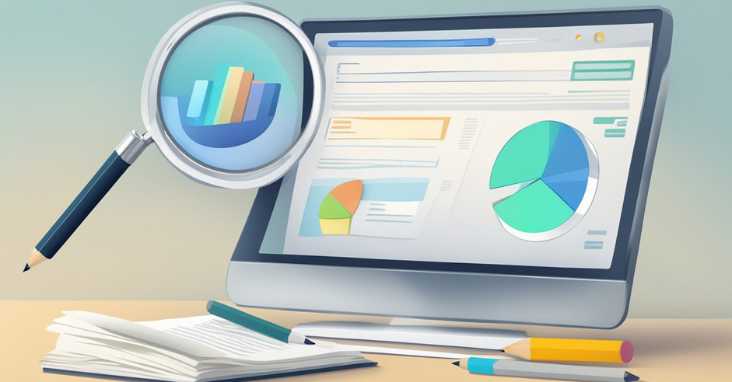
How does data enrichment improve data quality?
Data enrichment is the process of enhancing raw data by adding additional information from external sources. By enriching data, businesses can gain a more complete and accurate view of their customers, prospects, and markets. This, in turn, can help improve data quality by filling in missing pieces of information, correcting errors, and providing more context.
What are the primary techniques used in data enrichment?
There are several techniques used in data enrichment, including data appending, data normalization, and data deduplication. Data appending involves adding new data fields to existing records, while data normalization involves standardizing data to a common format. Data deduplication, on the other hand, involves identifying and removing duplicate records from a dataset.
In what ways does data cleansing differ from data enrichment?
Data cleansing is the process of identifying and removing inaccurate, incomplete, or irrelevant data from a dataset. While data enrichment involves adding new data to existing records, data cleansing focuses on improving the quality of existing data. Both processes are important for ensuring accurate and reliable data.
Can you provide examples of how data enrichment is applied within Salesforce?
Salesforce, a popular customer relationship management (CRM) software, offers several data enrichment features. For example, the Data.com Clean tool can help identify and remove duplicate records, while the Data.com Prospector tool can help find new leads and contacts. Salesforce also integrates with several third-party data enrichment providers, such as ZoomInfo and InsideView.
What role does artificial intelligence play in data enrichment processes?
Artificial intelligence (AI) can play a significant role in data enrichment processes by automating the identification and extraction of relevant data from unstructured sources, such as social media and news articles. AI-powered tools can also help identify patterns and relationships within data, which can be used to make more informed business decisions.
What should be considered when selecting a data enrichment service provider?
When selecting a data enrichment service provider, businesses should consider factors such as the provider’s data sources, data quality, pricing, and customer support. It is also important to ensure that the provider complies with relevant data privacy regulations, such as GDPR and CCPA. A helpful resource for evaluating data enrichment providers is G2, a software review site that provides user ratings and reviews of various data enrichment tools.
For more information on data enrichment, check out this article on Techopedia.